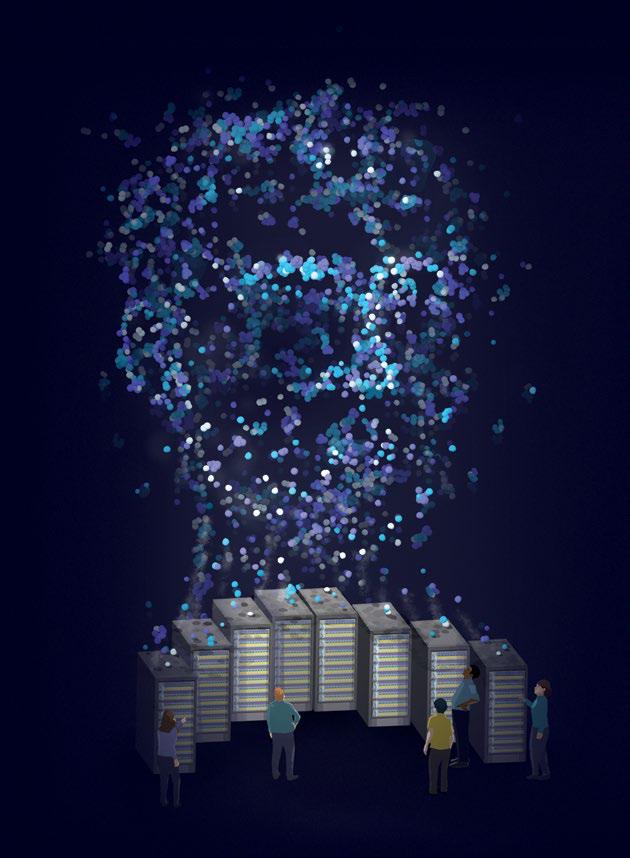
NOWADAYS, DEPLOYING ARTIFIcial intelligence no longer guarantees a competitive edge. What truly sets companies apart is access to diverse, extensive, high-quality data that enhances their AI system's performance compared with that of their competitors.
But concerns over data privacy can limit the use of unique, relevant data for analysis.
This problem can be alleviated by means of privacy-preserving federated learning. This technique, in combination with a special type of encryption, enables an AI model or any other type of algorithm to be trained using data from multiple, decentralized servers controlled by different organizations - all while respecting the privacy of the individuals or organizations whose data is being used for the training.¹ Simply put, federated learning entails sending the algorithm to the data rather than sending the data to the algorithm.
This is how Switzerland-based Zurich Insurance Group was able to improve a predictive algorithm with data from Orange, a British telecommunications company. Using a commercial federated learning platform, Zurich's algorithm could be trained, and its predictive capabilities improved, without the need for Orange to release any data. The collaboration led to a 30% improvement in the AI systemâs predictions, which translated into a significant revenue increase for Zurich. For Orange, it represented a new way of monetizing its data while still preserving its privacy.
Federated Learning Across and Within Industries
Real applications of federated learning are now rapidly emerging as organizations search for more data on which to train the AI systems they hope will deliver competitive advantage. For example, a large bankâs credit unit used the approach to fine-tune its algorithm for predicting loan defaults, using data owned by one of the largest global telecommunications companies, and improved prediction accuracy by about 10%.
ãã®èšäºã¯ MIT Sloan Management Review ã® Winter 2025 çã«æ²èŒãããŠããŸãã
7 æ¥éã® Magzter GOLD ç¡æãã©ã€ã¢ã«ãéå§ããŠãäœåãã®å³éžããããã¬ãã¢ã ã¹ããŒãªãŒã9,000 以äžã®éèªãæ°èã«ã¢ã¯ã»ã¹ããŠãã ããã
ãã§ã«è³Œèªè ã§ã ?  ãµã€ã³ã€ã³
ãã®èšäºã¯ MIT Sloan Management Review ã® Winter 2025 çã«æ²èŒãããŠããŸãã
7 æ¥éã® Magzter GOLD ç¡æãã©ã€ã¢ã«ãéå§ããŠãäœåãã®å³éžããããã¬ãã¢ã ã¹ããŒãªãŒã9,000 以äžã®éèªãæ°èã«ã¢ã¯ã»ã¹ããŠãã ããã
ãã§ã«è³Œèªè ã§ã? ãµã€ã³ã€ã³
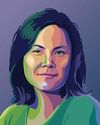
Ask Sanyin: How Do You Build for an Unpredictable Future?
While the pandemic was a wild ride of uncertainty for me and many of my peers in leadership, it feels like we never regained our footing.
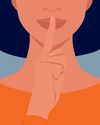
What You Still Can't Say at Work
Most people know what canât be said in their organization. But leaders can apply these techniques to break through the unwritten rules that make people self-censor.
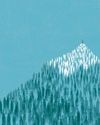
Make Character Count in Hiring and Promoting
Most managers focus on competencies when evaluating candidates but itâs character that will transform the DNA of the organization. Hereâs how to assess it.
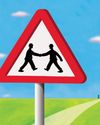
Why Influence Is a Two-Way Street
Managers achieve better outcomes when they prioritize collaborative decision-making over powers of persuasion.
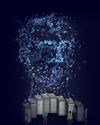
Know Your Data to Harness Federated Machine Learning
A collaborative approach to training AI models can yield better results, but it requires finding partners with data that complements your own.
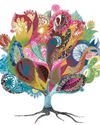
How Integrating DEI Into Strategy Lifts Performance
Incorporating diversity, equity, and inclusion practices into core business planning can provide a competitive edge.
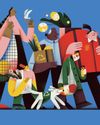
The Myth of the Sustainable Consumer
Companies that understand the different kinds of consumers for sustainable products can market to them more effectively.
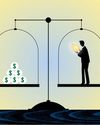
A Practical Guide to Gaining Value From LLMs
Getting a return from generative AI investments requires a systematic approach to analyzing appropriate use cases.
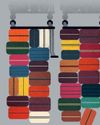
Improve Workflows by Managing Bottlenecks
Understand whether process or resource constraints are stalling work.
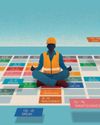
Craft Schedules That Work for Everyone
Business leaders can improve retention and business performance with schedules that make sense for workersâ lives.